Chapter 2
11 Types of cognitive biases to avoid in user research
Understanding different biases is the first step to ensuring they don't infiltrate your UX—in this chapter, we'll cover the 11 most common cognitive biases, including how they impact UX research, and what signs to look out for.
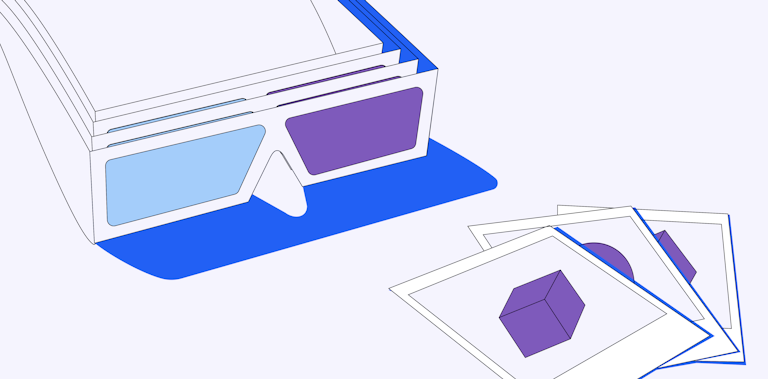
Cognitive biases are effectively your brain’s mental shortcuts to process information faster and increase the efficiency of decision-making. While the mind uses these effects to try and increase efficiency, cognitive biases are comprised of assumptions, making them unreliable or warped.
They live deep within your subconscious, and although humans use biases all the time without realizing, they can have some negative consequences, like making inaccurate judgments on information.
Cognitive biases can heavily influence the accuracy and effectiveness of the UX research process, as they may influence how you prepare your study, and view and interpret the data collected. If user research is about uncovering the raw truth, cognitive biases are the antithesis of this.
1. Confirmation bias
Confirmation bias is our tendency to assign more value to—and try to obtain—information and ideas that match those we already believe, thereby confirming our existing stance or suspicions. This bias also means we’re more likely to share that information over something else, as a way to articulate that we’re correct and back up our position. Similarly, it increases the likelihood of us subconsciously skipping over or outright dismissing information that goes against our belief or understanding.
Example of confirmation bias
Imagine you’re conducting user research on popular social media apps. You personally believe that
Instagram will be the favorite—that’s what you and most of your friends prefer, so it makes sense to you. In your research, some participants share they too prefer Instagram, while equally as many report Facebook as their favorite.
Instead of sharing the data that both apps are equally preferred, you focus your findings around the positive sentiments Instagram received and briefly skim over the feedback provided about Facebook. In doing this, you highlight one side of the findings (your side), and encourage others to agree, even incidentally incurring the framing effect.
2. Framing effect
The psychological bias behind leading questions, the framing effect is important to be mindful of in all areas of UX research. From first scoping your user research questions, to creating your interview guide, and sharing insights back to stakeholders—how you frame your questions and insights can be perceived radically differently, depending on the narrative you tell.
The framing effect happens when information is presented in either a positive or negative light, leading others to perceive that information in a particular way (i.e. put a ‘frame’ around it).
Example of framing effect
One way the framing effect could come into play is if you’re conducting research via a usability test to evaluate two competing marketplace concepts—you have each test participant complete a series of usability tasks on both concepts, before having a discussion to understand how they compared the two options.
During the session you observe that option A seemed to make more sense for the participant, and they struggled more using option B, even though option B shared more information about the marketplace services. After the usability tasks, you say “I could see option A worked better for you, tell me more about that?”.
By saying “option A worked better for you”, you’ve framed option A in a positive light and presumed which the participant preferred. From this point on, it’s likely the participant would predominantly share only (or mostly) positive feedback about option A, and negative feedback about option B.
3. Anchoring bias
This cognitive bias occurs when we find something to refer our decisions and/or reasoning back to—the anchor. The anchor is usually the first piece of information we receive, as it forms our understanding of the topic. Depending on what that is, this can become challenging if the initial anchor isn’t the best or most relevant option, as it can throw user research off course.
For example, anchoring often happens during negotiations like determining a salary or selling a car: once the first price is shared, this becomes the anchor for any future discussions or decisions, making it difficult to negotiate a lower price even if both parties would have accepted it.
Example of anchoring bias
If you’re conducting a study to gauge what people would pay for a new subscription service, you might start by asking “Would you pay $9.99/month?”. However, you’ve now ‘anchored’ them to thinking a subscription service is worth $9.99/month, even if previously they thought it was only worth $4.99. This also means they’ll likely refuse to pay more than $9.99, even if, in reality, they may have been willing to pay $11.99.
4. Serial-position effect
Also known as the primacy/recency effect, this phenomenon occurs because people are more likely to remember information at the beginning (primacy) and/or ending (recency) of a list, compared to information from the middle.
This is a common psychological effect, due to the amount of stimuli—and therefore information retention needed—at either end of an experience, compared to the middle. Studies on memory and recall have shown that participants tasked with remembering a list of items often score higher on remembering items from the beginning or end of the list.
Example of the serial-position effect
You’re creating a survey for a car-sharing app, to better understand user needs. One of your questions asks the respondent to share what features are most important to them when choosing a car to drive. The list of options you’ve provided consists of 30 unique answers. After receiving the responses, you’re likely to notice a trend where most people have chosen the options at the beginning or end of the list, even if these answers don’t necessarily align with the rest of their feedback.
5. Clustering illusion
Clustering illusions occur when we think we see patterns in data, simply because of how the data is presented, i.e. in groups or occurring in a close timeframe. Our brain has been designed to identify patterns as a way to be more efficient and speed up decisions, and therefore underestimates the amount of variability data has. However this can mean labeling something as a ‘pattern’, when in fact it is random information, where no pattern exists.
Example of clustering illusion
A common way this cognitive bias presents is in business trends. For example, you share a research report with your stakeholders regarding reasons for churn, identifying that 0.5% of users attribute price as their reason for leaving. Another colleague catches wind of your research and also believes that price is a big contributor to why people leave the platform, because the day before they received this feedback from a customer. Others start to gather their insights on the topic, and everyone can recall hearing similar feedback.
However, after a full investigation, you learn that this 0.5% only summates approximately 10 people a year, compared to the remaining 99.5% of customers who cite other reasons for leaving the platform. These anecdotes and data points started to look like a pattern to the team because of how they were presented, but in reality, the big picture was not that alarming.
6. Empathy gap
The empathy gap is an emotional effect that highlights our tendency to dismiss how our emotional state can influence our behavior and the decisions we make. This is a very common bias, where we overestimate how impartial or emotionally ‘neutral’ we may be. It’s especially prevalent in a world where we spend much of our time using technology, communicating virtually, and being somewhat disconnected from our emotions due to multiple distractions simultaneously.
An example of empathy gap
An example of the empathy gap is if for months you’ve been preparing for your first career development meeting at work—this meeting is really important to you, as you’re working towards a promotion. During the meeting, it doesn’t go as well as you’d hoped, and you leave feeling disheartened.
About an hour later, you have a user interview for a research project: during this interview, you rush through the questions and struggle to listen. As a result you miss several opportune moments to ask follow-up questions. Others observe that the interview felt rushed, but you struggle to understand that there was anything wrong, let alone identify why that was.
7. False-consensus bias
A particularly common cognitive bias when working on product research, the false-consensus bias causes people to believe that their personal experiences are more common than they actually are. It also heavily influences people to assume others will agree with us, particularly if it’s a subject (or product) we are familiar with or working closely on.
This bias might happen if you work for a technology company and use that technology in your day-to-day life, or if you’ve been working on a new product release and are then asked to test it yourself. The biggest way this bias can be damaging is when individuals start to believe that people with different opinions or experiences to theirs are incorrect, and so disregard their input.
An example of false-consensus bias
You work for a travel service, and are in a stakeholder workshop to plan user research activities for the next quarter. There’s a list of potential areas to research and prioritize, and a stakeholder
expresses the main issue they personally have when traveling is leg room. Someone notes that user feedback points to luggage space as a more common frustration among users, however the first stakeholder insists leg space is more urgent to focus on, as they travel frequently and often struggle with this issue, so others must do too. In the end, the team focuses on leg room but noticed little change in overall user satisfaction when running a follow-up survey.
8. Peak-end rule
The peak-end rule is a phenomenon that shows the most memorable part of an experience happens where the most emotion is felt—this is referred to as the ‘peak’. The peak is dependent on context, and often coincides with the end of an experience. You can think of it like the climax of a story—the highlight or most emotionally-charged moment.
Because of the effect emotions have on memory consolidation (our brain’s act of committing something to long or short term memory), our memory is more reliable at these points; making the peak and end a more tangible memory, easier to recall, and of higher emotional impact. This is why we often remember extreme positives and negatives more clearly than the middle or ‘okay’ part of an experience.
This peak-end rule can work in tandem with the serial-position effect, where a highly emotional moment occurs in the middle of an experience, and can boost one’s otherwise low memorability of the middle section.
An example of the peak-end rule
Think about the last time you filled out a lengthy reservation request form. Now imagine there was an error in the form at the end of the process, just as you were about to hit ‘send’. According to this rule, you would have a strong memory of this specific moment, because of the emotional impact (frustration) it had on you. In this case, the memory would be cast in a negative light due to the frustration, and the ‘peak’ would be marked as this moment.
9. Question order bias
This cognitive bias denotes how placement of questions can influence results, depending on how you position them. This bias can be particularly prevalent in UX research methods like user surveys, where people have a tendency to respond to a question by simply choosing the first option that is presented to them. Similarly, question order bias can occur in a live interview, when a previous question influences how participants respond to subsequent questions, simply because of the design of the questions.
An example of order bias
Consider you’re running a survey to understand people’s video entertainment preferences—the goal is to decide which companies to partner with to increase signups. An example of order bias would be asking “1. Which Disney movie is your favorite?” and “2. What’s your favorite movie?”.
By the first question being focused on Disney, it is affecting how the second question is answered, because (particularly if the respondent is unsure on their favorite movie) they are primed to think of Disney.
As humans, we are innately predisposed to want people to like us. We want to be seen in a positive light, we want to be admired and desired, and avoid feeling ashamed, embarrassed or worthless. This can mean social desirability bias occurs, where people respond in a way that they believe (consciously or not) to be more socially acceptable and preferable.
A common example of social desirability is when people answer personal questions, such as number of romantic partners, or if they’ve ever committed a crime. In this sense, social desirability bias is heavily influenced by societal and cultural norms and expectations.
During research, this might manifest as participants responding to interview questions with an answer they think the interviewer wants to hear, in a way that will make the interviewer like them, or avoiding disclosing information that they deem embarrassing or inappropriate. However, this bias can lead to people providing answers that aren’t an accurate representation of how they really feel, but rather their interpretation of what they believe would make them seem likable.
An example of social desirability bias
One way social desirability bias could occur is if you were interviewing a user to assess an app post-launch. You ask questions about how understandable and usable the app is. Your participant insists they like the app and shares a lot of positive feedback, even when they start to clearly struggle with some of the usability questions.
Despite obvious flaws in the app, the interviewee doesn’t want to cause a fuss or upset the interviewer, so insists the app is better than others they’ve used. This bias is why it’s extremely important to watch what people do, and put more weight on behaviors (particularly of those in an unmoderated test), rather than just words.
11. Fundamental attribution error
Last on our list is a bias that focuses on the individual as the reason for a situation carrying out as it does, rather than the situation or circumstances around it. For example, instead of thinking that there might be extenuating circumstances, or a number of factors to explain an outcome, the blame is put on an individual—often a specific personality trait or who they fundamentally ‘are’.
An example of fundamental attribution error
In a usability test a participant struggles to find the purchase path on an e-commerce website. During the user testing, the researcher is acting frustrated and impatient with the participant, because they are not finding the purchase path. Understandably, the participant becomes increasingly nervous, continuing to struggle with the test.
After the session, stakeholders review the test and agree the participant struggled because they must have little experience with e-commerce websites. It doesn’t cross their minds to consider that the researcher’s behavior would have impacted the participant, or there might simply be challenges with the user interface—they instead put the blame directly on the user.
Avoid user research bias with Maze
Awareness of cognitive biases is step one of the solution to banish user research bias, but you don’t have to go it alone. There’s many UX research platforms out there which are designed to conduct objective, accurate research, and you can employ tools like usability testing templates to help keep research fair and unbiased. Now you know what biases to look out for, and how they can show up in UX, it’s time for chapter three, where we’ll dig deeper into how you can intentionally block your own cognitive biases from trickling down into your research.
Frequently asked questions about types of cognitive biases in user research
What are the types of cognitive biases in user research?
What are the types of cognitive biases in user research?
There’s over 180 types of cognitive biases in user research—including common biases like the false-consensus effect, social desirability, framing bias, confirmation bias, and the peak-end rule. When it comes to UX research in particular, it’s important to look out for cognitive biases that can impact your research questions, results analysis, stakeholders, researcher/interviewer, and participants.
How many types of cognitive biases are there in UX?
How many types of cognitive biases are there in UX?
It’s estimated there’s over 180 types of cognitive biases in UX—which ones show up can vary depending on the context; who you’re working with, what your product is, and even where in the world you are. When working in user experience, there’s a few common biases to look out for.
- False consensus bias: The belief that your behaviors or opinions are more common (and likely to be shared) than they might actually be.
- Primacy recency effect: A psychological phenomenon that occurs because we are often more likely to remember information presented at the beginning or end of an experience.
- Frequency bias: The belief that something is more common than it actually is, as a result of you recently starting to notice or pay attention to it.
- Social desirability: The tendency to respond or behave in a way you believe will be favorable to another.
- The peak end rule: The phenomenon showing that the most memorable part of an experience is wherever the most intense emotion is felt i.e. the ‘peak’. This affects people’s ability to accurately recount the entire experience end-to-end.
- Framing effect: The occurrence where responses are biased depending on whether the information was presented in either a positive or negative light.
- Confirmation bias: This bias is in action when we seek out and assign value to information that confirms our already-formulated beliefs.
10. Social desirability bias