Chapter 3
Customer insights analysis: How to turn data into decisions
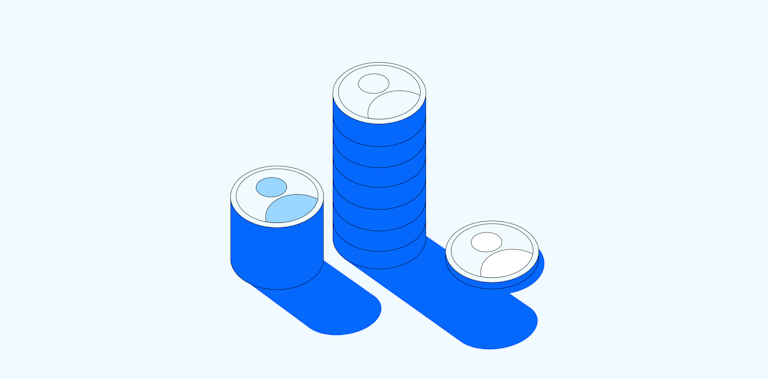
Customer insights analysis helps you make sense of the data you’ve collected, giving you a clear picture of your customers’ needs, preferences, and motivations throughout the customer journey. It’s these insights that give you the information you need to improve your product’s customer experience.
In this chapter, we cover how to conduct customer insight analysis and measure the success of your customer insights strategy with set customer-focused metrics. Let’s start by clarifying the types of customer data you’ll see.
What are the types of customer data analysis?
Quantitative and qualitative research data are the two main types of customer data analysis. Quantitative data typically answers the question ‘What?’, whereas qualitative analysis looks at ‘Why?’. Using these two types of customer data analysis together (mixed methods) gives a comprehensive overview and deeper understanding of customer sentiment and experience.
Quantitative data analysis: Finding your ‘what’
Quantitative data analysis is the process of interpreting numerical data to identify patterns, trends, correlations, and relationships within your responses. It relies on statistical methods to give you objective and measurable findings.
Some of the customer research methods that require quantitative data analysis include close-ended surveys with customer metrics like CSAT and NPS, and behavioral data from usability tests such as A/B testing, web or product analytics.
For example, let’s say you’ve conducted a closed-ended UX survey to see what your customer base thinks about your platform’s newly integrated AI tool. Replies begin rolling in, and you start tallying up the responses to get the percentage of users who are happy (or unhappy) with the new AI tool.
You find that 67% of your customers surveyed find the AI tool “unhelpful” or “hard to use” in some way. Answers to another question indicate that 85% of users don’t regularly use the AI tool to help them with their jobs-to-be-done.
These insights signify that there are usability issues with your new AI tool. After analysis, you can conduct further UX research to identify why users don’t find the AI tool helpful and apply findings to build a better user experience.
Qualitative data analysis: Finding your ‘why’
Qualitative data analysis is the process of interpreting non-numerical data to understand customer experiences, motivations, and opinions within your responses. It helps provide you with the meaning behind the data—the why behind quantitative data’s what—giving you a more holistic look at what guides your customer’s choices.
Some common customer research methods that require qualitative data analysis include user interviews, focus groups, market research, and online reviews from social media platforms. Typically, the data sets for qualitative studies are much fewer, and the process involves thematic analysis to identify key, overarching themes and patterns within your data.
Let’s return to our previous example. Say you’ve conducted a round of customer interviews to better understand why customers don't find the AI tool typically helpful. After reviewing interview transcripts, you see a common theme emerging. Most customers consider the functionality unhelpful because of its constant display of example prompts, making it difficult to operate effectively.
Based on these insights, you brainstorm solutions with your design team. Given that you know that users find that example prompts get in the way, you settle on removing that messaging from your tool. However, you also add a ‘Show prompts’ button just in case users need any help.
How to analyze customer feedback to uncover customer insights
Regardless of the type of analysis you’re conducting, you’ll need a set of steps to ensure you get accurate and actionable insights. A standardized analysis process gives you a reliable framework for turning data into insights for your team. Here are four steps to nail your next customer feedback analysis.
1. Collect your customer responses
Like any user research analysis, for customer insight analysis to be efficient, you need to ensure that all your data is well-organized in one place.
If you’re analyzing your quantitative data manually, begin pulling out responses from your research platform and placing them in an external repository, like Google Sheets or Excel. Doing so gives you a better overview of your data and ensures you can manage it accurately.
In the case of qualitative data, keep screen recordings and interview transcripts in a centralized database, such as Google Drive or Notion. Keep in mind that if you’re not using a tool that automatically transcribes your interviews, you’ll have to do so manually.
A comprehensive UX research tool can be a big help here, as it removes the need to manually analyze, export and manage your data. For example, Maze AI offers video transcripts, automated highlight tagging, AI-assisted thematic analysis, and Automated Reports to ensure all your responses are consolidated in one interface, making it easier and quicker to analyze results.
💡 Pro tip
If you haven’t already, consider creating a research repository where you can store your data (and your insights once you have them) to revisit during future initiatives. This will provide you with context and help you track your progress with insights.
2. Review and clean up your data
Not all of your data is useful, and keeping corrupted and unhelpful responses in your repository can lead to inaccurate analysis and skewed results.
To ensure your quantitative data is completely clean, remove responses containing:
- Partial responses: Participants may leave certain questions unanswered due to technical difficulties or fatigue.
- Straightlining: A phenomenon that appears with multiple choice questioning. If you’ve received straight ‘C’s to each of your multiple-choice questions, your participant likely wasn’t answering honestly, making the data disingenuous and unusable.
- Overly fast responses: Consider the time participants take to give their responses. For example, if a CSAT survey took two minutes for one customer but took seven for other participants, the customer who spent less time was likely trying to finish as fast as possible.
- Outliers: These data points deviate dramatically from the rest of your answers on a subject. In certain cases, these deviations are just unique answers. Other times, they indicate answers participants provided at random. To identify outliers, look for contradictions within the same respondent’s data.
For qualitative data from interviews and focus groups, make sure your transcription follows a standardized format with timestamps, paragraphs, and speaker labels. This will ensure that your data is easy to read and review when performing analysis.
3. Quantitative data: Perform statistical analysis
If you’ve collected data through a quantitative research method like a closed-ended customer experience survey, the next step is to perform statistical analysis. Begin by calculating important stats such as averages and percentages, and placing answers within your spreadsheet. As patterns emerge, you’ll begin to identify trends in user sentiment, customer satisfaction, and usability.
Statistical analysis can be a resource-intensive process. We recommend using a tool like Maze to get the job done. With automated analysis, you can analyze data almost as quickly as it comes in, making it easy to uncover insights from Feedback Surveys and Live Website Testing.
Kirsty Finlayson, Director of Marketing at Chameleon notes the value of having the right tool is:
“If you have a lot of insights and responses to analyze, having a tool with native AI summaries can help you out here. I find the hardest part of analysis is starting, and having a short overview speeds things up a lot. Most survey/insights tools have this built into their dashboard now.”
4. Qualitative data: Conduct thematic analysis
If you’ve selected a qualitative customer research method such as Interview Studies or focus groups, you’ll need to conduct thematic analysis to extract insights.
Begin by reading through interview or focus group transcripts and assigning codes to any patterns that repeat themselves. Codes should act as a brief summary of any point of interest.
For example, a customer might say:
“I don’t like the annoying AI feature, and it’s really spoiling my experience with the product. The pop-up seems to follow me everywhere.”
Based on the opinion above, the relevant codes would be:
- Negative experience with the AI feature
- AI feature placement
Codes will help you identify prevailing patterns within your data set. Group them into relevant, overarching themes as they emerge, and label each with a subject matter.
Finally, review which themes and codes repeat themselves the most to find insights. For example, customers complaining about AI feature placement under the theme AI feature usability gives you actionable insights on the functionality.
Once you’ve analyzed your data, you can apply insights to your product to improve the customer experience. Next on your list is checking that these insight-driven updates have been successful.
Metrics for monitoring the success of your customer insights initiatives
New products or new features implemented as a result of customer insights need to translate into tangible business outcomes to be considered successful. The only way to know if your insights have improved the customer experience is by measuring and tracking your product’s performance and UX metrics after you’ve introduced insight-backed solutions.
Here are the best metrics for benchmarking and monitoring your customer insight success.
1. Customer satisfaction (CSAT) score
The CSAT score is a key performance indicator (KPI) that measures your customers’ overall satisfaction with your product or service.
Measuring it tells you how well you meet customer expectations while helping you identify potential ways to improve the customer experience. After applying insights to your product, measure CSAT once every six months, and then monitor progress.
To calculate CSAT, use the formula:
CSAT = (number of satisfied ratings as defined by your rating scale / total number of ratings) x 100
2. Customer effort score (CES)
The CES tells you how easily customers can interact with your product—whether that’s finding guidance, completing tasks, or resolving issues. Regularly monitor this benchmark if your insights have led to changes that could affect your product’s usability metrics.
CES can also help you quickly identify any customer friction points you’ve missed, helping you determine the next customer experience problem to address.
To find your CES, you’ll need customers to complete a survey triggered after completing a task with your product. You then use Likert scale questioning to gauge how easily they could complete the task.
For example: “How easy was it to sign up for our service?”
- 1 - Very difficult
- 2 - Difficult
- 3 - Neutral
- 4 - Easy
- 5 - Very easy
To calculate CES, use the formula:
CES = sum of responses / total number of responses
3. Net Promoter Score® (NPS)
Measuring your NPS helps you assess customer loyalty and understand the likelihood of existing customers suggesting your product or service to others. It also helps you quickly identify which customers are happy with their overall experience (those likely to share your product with others) and who are at risk of churning.
Determining NPS after you implement insight-driven updates requires asking customers a single question:
“How likely are you to recommend our company on a scale of 1–10?”
Organize your answers into three groups:
- Detractors: Answered 0–6
- Passives: Answered 7–8
- Promoters: Answered 9–10
Finally, calculate the percentage of your answers with the formula:
NPS = percentage of promoters - percentage of detractors
4. Customer retention and churn rate
Customer retention rate is a crucial KPI that measures how many customers you keep during a set period. The churn rate is the inverse—it indicates how many customers leave during a set period. Both metrics are important for understanding overall customer satisfaction and loyalty.
To calculate the customer retention rate, follow the formula:
CRR = (customers retained over a set period / total customers at the start of the period) x 100
To understand your customer churn rate, use the formula:
CCR = (customers lost over a set period / total customers at the start of the period) x 100
5. Bounce and conversion rates
After applying new insights to your digital experience, you’ll want to monitor how well your solutions perform with potential new customers through bounce and conversion rates. These two metrics are especially important when you’ve made major changes to your website’s or platform’s UX.
Bounce rates help you measure the percentage of people who leave your website after viewing just one page. A high bounce rate indicates that potential customers haven’t found what they're looking for.
To calculate bounce rates, follow the formula:
BR = (single page sessions / total number of page sessions) x 100
Conversion rates, on the other hand, measure the percentage of users who have completed a desired action using your digital experience or service. These could be sign-ups, onboarding, or purchases.
To calculate conversion rates, use the following formula:
CR = (number of conversions / total number of visitors or interactions) x 100
Leverage customer insights with comprehensive customer feedback analysis
You can’t get valuable customer insights without analysis. Whether you’ve conducted a customer experience survey or you’ve talked to customers in a focus group, interpreting your data plays a crucial role in getting actionable insights. As important as analysis is, it’s also a resource-intensive process.
Tools like Maze can help streamline customer insights analysis with a comprehensive suite of research analysis features. From key statistical insights via usability tests, to AI-powered interview analysis, Maze is the analysis copilot for any CX program.
Frequently asked questions about customer insights analysis
What is customer insights analysis?
What is customer insights analysis?
Customer insights analysis is the process of examining and interpreting data collected from research to understand customer behavior, preferences, pain points, and needs. Through insights analysis, you turn the responses you’ve collected into actionable findings your team can use to drive decision-making and improve the customer experience.
What does a customer insights analyst do?
What does a customer insights analyst do?
A customer insights analyst reviews customer data to extract insights that can help make more informed decisions. They collect and analyze data to identify existing issues and new opportunities.
What is a customer insights example?
What is a customer insights example?
A customer insights example could be finding out that customers aren’t happy with a recent update. It could also be uncovering that 90% of customers don’t use over 50% of your offerings. Customer insights vary greatly, but it applies to any insight you have on your customers, how they feel, and how they think.